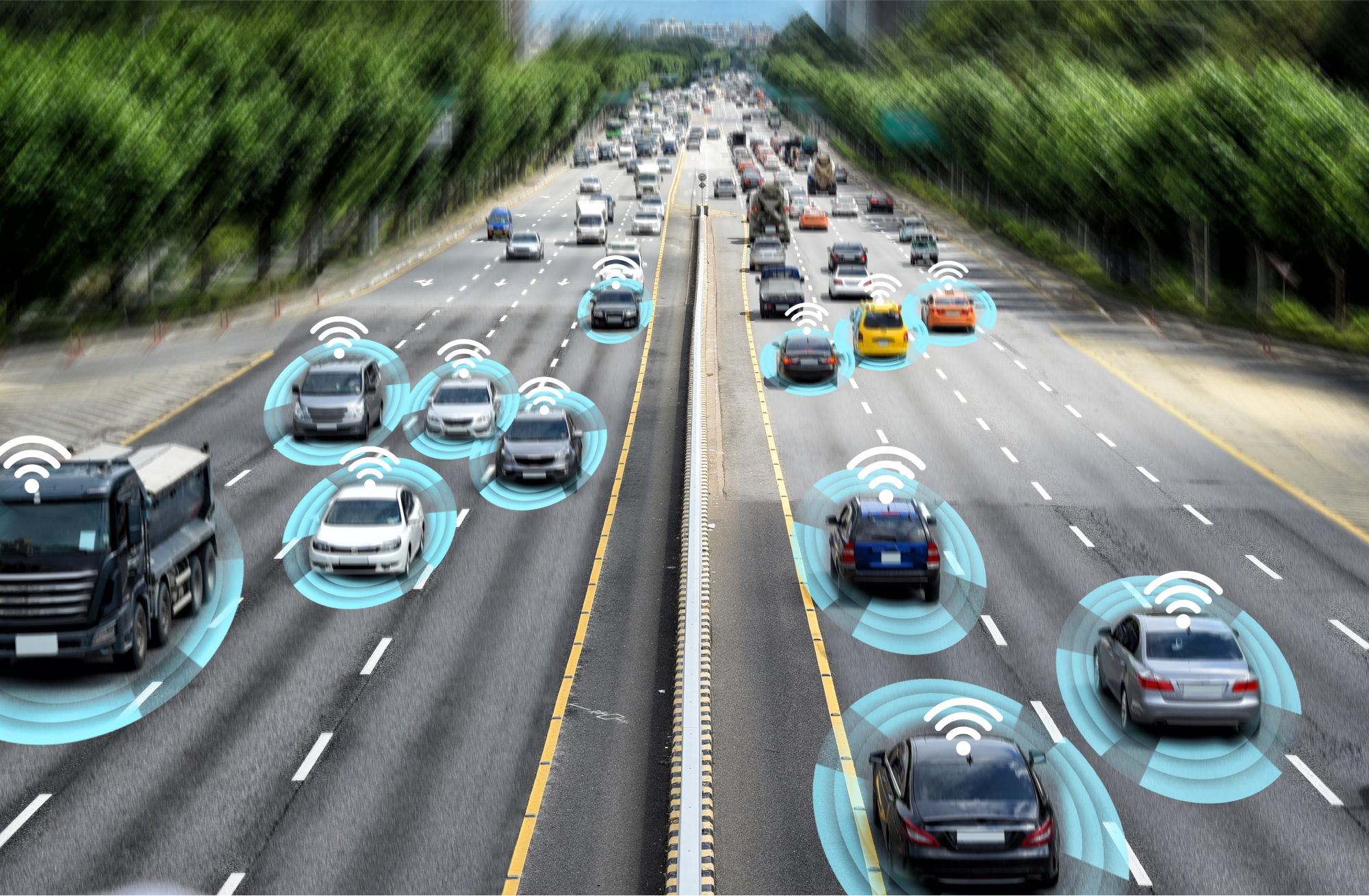
The Report
1.2 Screening and classification
1.2 Screening and classification
The output of the identification tasks led to the compilation of 1,599 candidate soft law programs. With this information in hand, the next step was to validate and classify them. The research team took a second look at each program to verify their compliance with the inclusion criteria. If they complied, the final step would be to extract information (see Figure 1 or a more detailed version of this figure is located in Appendix 3).
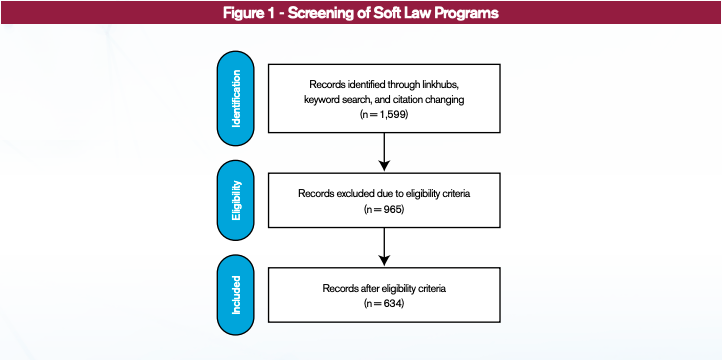
1.2.1 Screening
Once identified, candidate programs were subject to an additional layer of scrutiny. A team member, who was not originally charged with locating a program, verified its compliance to the project’s inclusion criteria. If it did not meet the three requirements, it was excluded from the database. This led to the elimination of about 60% of programs.
Programs were eliminated from the database for one of six reasons (see Table 1). About 600 programs are articles in the popular or academic press that did not include a soft law element [14]–[16] or could not be placed under any soft law category [17], [18]. Another 121 programs passed the threshold of classifying as soft law, but were either not related to AI [19], [20] or published in 2020 [21], [22]. The final two categories are composed of documents classified as recommendations, except that they did not create substantive expectations of action on any stakeholder. For instance, 171 documents were authored by non-profits that targeted recommendations to parties with whom there was no apparent relationship [23], [24]. Similarly, entities in the private sector published 80 documents selling their services (e.g. consulting firms) or directing recommendations to audiences of potential consumers and the public [25]–[27].
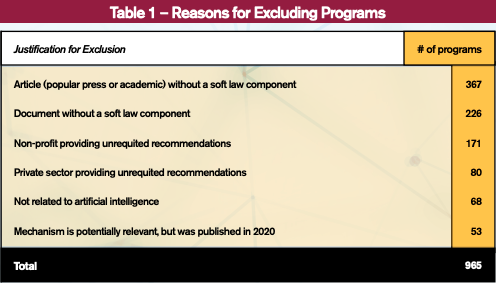
1.2.2 Classification
One of the project’s objectives is to detect trends in how organizations employ soft law in the management or governance of AI. To accomplish this, the core research team consulted similar efforts [1], [2] and brainstormed on what information could be extracted from these programs. This led to the differentiation between two types of data: variables and themes. Variables provide information on how a program is organized, functions, and its general characteristics. While themes communicate the subject matter discussed within its text. Overall, our project classified each program with up to 107 variables or themes (see Table 2).
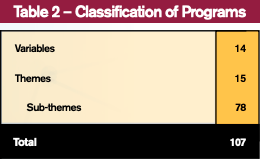
The variables and themes in this analysis were developed via a pilot exercise with 35 randomly-selected screened-in programs. Each program was assigned to two individuals and their task was to examine the type of information that could be extracted. The pilot resulted in the creation of 14 variables and 15 themes.
Variables were generated through a consensus-driven process. They provide detailed information on how a program functions, its jurisdiction, and who participates in it. Themes were inspired by the work of Fjeld et al. [1]. In this sense, team members were asked to partake in a pile-sorting exercise [2]. Pile sorting is a process meant to elicit common attributes of information by bundling it into piles and discussing their shared characteristics until an agreement is reached. In this project, team members were asked to generate labels that described the text within a program. Subsequently, these labels where discussed and merged into groups until a consensus of a general taxonomy of themes was reached. To operationalize this process, one individual was tasked with classifying the text of a program, while a second validated their work (see Appendix 4 for the inventory of variables and themes).
When the classification of the 634 programs concluded, a second pile-sorting exercise was performed to generate sub-themes. In this exercise, two individuals generated labels pertaining to a representative sample of 20% or at least 20 excerpts from each of the 15 themes. This information was used in a session where three team members met virtually to pile-sort the labels. The outcome was the creation of 78 sub-themes. Subsequently, one project team member was assigned to label a theme’s text into its respective sub-themes.