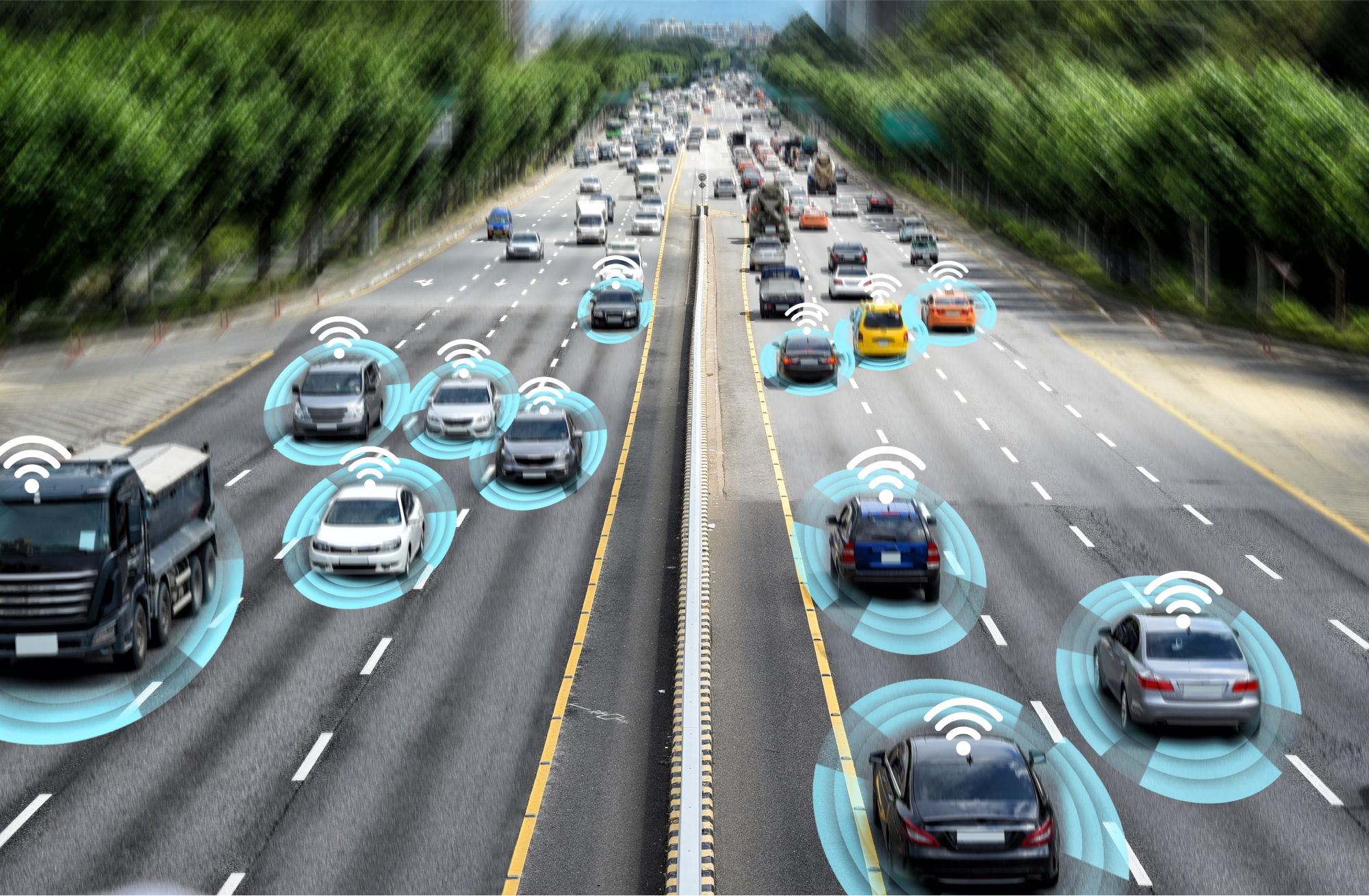
The Report
Summary
Soft law is defined as a program that sets substantive expectations, but is not directly enforceable by government. Because soft law is not bound by a geographic jurisdiction and can be developed, amended, and adopted by any entity, it will be the dominant form of artificial intelligence (AI) governance for the foreseeable future. The objective of this document is to compile and analyze global trends on how this governance tool is used by government, non-profits, and the private sector to manage AI’s methods and applications.
Inspired by similar efforts, this document contains a scoping review of AI soft law programs. Our process was divided into three steps: identification, screening, and classification. Our identification of programs began by establishing eligibility criteria. All programs had to: 1) conform to the definition of soft law, 2) emphasize the governance or management of a method or application of AI, and 3) were published by December 31st, 2019. These criteria made it possible to detect relevant programs through one of three methods that were implemented in a parallel manner. We found and mined over 80 linkhubs, resources that aggregate programs. We performed 370 keyword searches that combined our soft law program typology with a diverse list of themes, applications, and methods related to AI. Lastly, every screened-in program was vetted to search for references to other relevant programs, efforts such as these are denominated citation chaining.
In the screening process, we verified each program’s compliance to the project’s eligibility criteria. Out of the 1,599 programs initially identified, 965 were excluded because they were deemed to be articles or documents without a soft law component, unrelated to AI, or published after our 2019 cut-off. The final step of the process involved classifying the programs. Through several pilot exercises and by adopting best practices from relevant research, we developed 107 variables and themes to describe the programs. Variables provide information on how it is organized, functions, and its general characteristics, while themes communicate the subject matter discussed within a program’s text.
Overall, we identified 634 soft law AI programs. Through our variables and themes, we were able to gather insights from this database. For one, the governance of this technology through soft law is a relatively new endeavor. Despite finding programs from the year 2001, over 90% of those in our sample were published between 2016-2019. Geographically, there appears to be limited diversity. The vast majority originate in countries classified as high income within Europe and North America. In the development of these tools, organizations appear to overwhelmingly prefer programs geared towards influencing the behavior of internal and external stakeholders, as opposed to those limited to internal stakeholders. At the same time, less than a third publicly mention enforcement or implementation tools meant to compel compliance with soft law program.
Despite having seven categories for classifying the type of soft law program, about 80% were labeled as principles or recommendations/strategy. This includes a list of 158 principles, one of the largest compilations dedicated to AI available in the literature. Our research also dispels the notion that soft law is the exclusive purview of industry self-regulation. Through the creation of governance triangles, we found that government entities led AI soft law development with a ~36% participation, followed by multi-stakeholder alliances with ~21%. One of the original contributions of this research is its classification of each program’s text. By harnessing our 15 themes and 78 sub-themes, we uncovered that general mentions of transparency, general mentions of discrimination and bias, and AI literacy are the most represented issues in our database.
Soft law is not a panacea or silver bullet. By itself, it is unable to solve all of the governance issues experienced by society due to AI. Nevertheless, whether by choice or necessity, soft law is and will continue to play a central role in the governance of AI for some time. As such, it is important to build-upon the lessons that emanate from this research to make soft law as effective and credible as possible so it can address the governance challenges of AI systems, including safety, reliability, privacy, transparency, fairness, and accountability.
The ultimate goal of this research project is to inform decision-makers with evidence, practices, and recommendations that can be harnessed to enhance soft law programs with the objective of improving the management of applications and methods of AI under their responsibility.